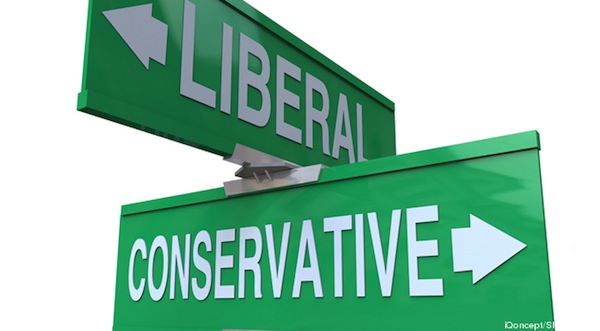
We live in very complex times. As much as we talk about integrated insights, it only seems to be getting worse.
If I sound a little bleak it’s because this statement has been shared ad nauseam: digital marketers are literally drowning in data.
Data sources range from anecdotal and trivial to scientifically precise, and span numerous systems both on premise, in the cloud, and property of third-party data providers. Using several approaches to arrive at a single truth (to within a reasonable variance, I might add) is extremely difficult.
Many share this conservative view on data democratization, believing that only data scientists and digital analysts should embark on these journeys into meaningful and rigorous analysis, while others argue that data should be liberated, free for all to use.
Debates on this subject usually happen in the upper echelons of an organization. The classic arguments surround data ownership and often pit marketers against technology, amongst other silos.
While there is plenty of material that analyzes the data ownership debate, data democratization involves the access, usage, and (more importantly) the decisions influenced by multiple groups conducting research and making recommendations to the organization. And while some points may only resonate with enterprise-level organizations, digital marketers and analysts alike working at small and medium-sized businesses would be prudent to recognize the challenges and maturity milestones introduced with scale.
Challenges surrounding data democratization abound, and every organization’s data practice must decide policies and responsibilities in order to remain impartial, so here are some arguments to keep in mind when deciding in which zone of the political spectrum of data democratization you fall.
Characteristics of Conservative data democratization:
- Data should be owned and analyzed by specific individuals or groups within their organization, forming a center of excellence.
- Strict governance over the collection, interpretation, and dissemination of information must be followed.
- Accountability resides within the data science group.
- Time is better spent studying data within the group than training those outside the group.
Pros of being Conservative:
- Dedicated resources officially responsible to producing high quality insights generally employ greater scrutiny when making recommendations, often analyzing a problem with multiple data sets and advanced modeling.
- Tighter controls over potentially risky data including confidential records, profiles, and information that gives the organization a distinct competitive or reputational advantage.
- Subject matter expertise often leads to data stewardship (i.e., greater accuracy and less susceptibility to multiple incongruent data trends and unexplained inconsistencies).
- Business value is easily exhibited for reinvestment in the team.
- Stakeholders spend less time churning on analyzing data, and more time on testing theories and implementing optimizations.
Consequences of being Conservative:
- Organizations with lower insights maturity lack the ability to ask the business questions that can affect positive change.
- Execution of analysis relies heavily on prioritization conversations, which can create friction.
- Achieving scale can be very difficult, especially with limited human resources available in the market.
- Strict insights practices may miss out on cost-reducing self-service opportunities by distributing lower-value work.
Characteristics of Liberal data democratization:
- Data should be owned by the organization and can be analyzed by anyone.
- Loose governance over the collection, interpretation, and dissemination of information.
- Accountability resides with the data user (i.e., the person or group reporting the data).
- Time is better spent training consumers across the organization to use the data themselves.
Pros to being Liberal:
- Distribution of information across working teams encourages entrepreneurial spirit, often benefitting smaller, more agile, or less regulated organizations.
- Usually many sources of data available at a user’s disposal due to looser controls over data collection.
- Data-savvy stakeholders tend to incorporate data analysis into their official mandate, making them less dependent on a centralized analysis team.
- If a dedicated analyst group exists, opportunities arise where external stakeholders or users of the data can be considered for positions within the insights team and vice versa.
- Lower value insights work can be pushed to self-serve capabilities rather than being deprioritized.
Consequences of being Liberal:
- Multiple data sources and data consuming groups lead to multiple versions of the truth, increasing churn and generally leading to less confidence in recommendations.
- If a dedicated analyst group exists, they may experience frustration with misinterpreted data across the organization.
- Less governance over the collection and usage of data may go violate privacy and regulatory controls.
- User experience may be negatively impacted with too many data collection methodologies.
- Redundant job functions across multiple groups can be more costly to an organization than a centralized insights team.
While it’s easy to preach one democratic approach over the other, the reality is that extremely conservative or liberal views rarely fit in the context of data democratization. Business leaders need to carefully weigh the pros and cons of data democratization to determine which approach benefits their organization the most.
Where do you and your organization land in the spectrum? Feel free to comment below!